Student Support uplift at Wintec using Machine Learning
To allow better targeting of at-risk students, whilst providing ongoing, statistical evidence of the efficacy of the student services, a machine learning model was produced.
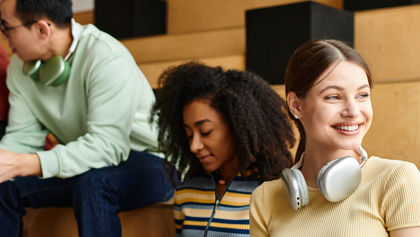
The Waikato Institute of Technology (Wintec), based in Hamilton (New Zealand), delivers high-quality training to approximately 13,000 ākonga/learners every year, who study more than 130 vocational courses across many fields from certificate to degree, postgraduate and master's level. Wintec provides numerous pastoral care and academic support services to students. This support has traditionally been inconsistent, and measurement of success has relied on anecdotal evidence.
To allow better targeting of at-risk students, whilst providing ongoing, statistical evidence of the efficacy of the student services, a machine learning model was produced. A scientific study was designed and scheduled, however due to un-anticipated changes in the business support strategy, Aware Group (an HSO company) postponed the trial to avoid consuming capital when the integrity of the results obtained could not be evaluated. The model was re-purposed to identify the most at-risk pre-degree programs’ and student cohorts; a function previously performed via basic numerical analysis of the prior years’ results.
Challenges
The existing support structure at Wintec has inefficiencies and is highly dependent on Tutor and Support Staff observation of students to note when students may be struggling.
Some of the issues with the existing system are:
- Reactive care – Much of the support structure requires the student to recognise they are experiencing trouble early enough for intervention to be effective.
- An amount of one-on-one time with students, which without at-risk targeting is expensive and inefficient.
- Bulk support for students, such as workshops, reach many students that are not at-risk in the hopes of supporting a few who do.
In addition, due to lack of visibility of effectiveness, it has been difficult to effectively determine the appropriate level of support.
Benefit of product
The developed model would have provided support staff with a database of specific students to contact, along with a gauge of the impact they could make, and evaluable results of how effective their interventions have been. Once evaluated as part of the scientific trial, this would provide the ability for management to gauge how many staff are needed. Improved services would also lead to better Maori/Pasifika completion rate parity.
The re-purposed use of the model designed to replace the basic numerical analysis for at-risk student cohorts and programs provides the support teams with focus as to which groups to embed staff in, and allows them to focus on groups have the most need. While not directly predicting the expected improvement, they will be able to provide to individuals, the model allows them to make better use of the resources they have.
Results, ROI and Future Plans
The anticipated results of the study were:
- Evidence of improved course completion rates and Maori/Pasifika parity.
- Statistics-based decision-making on resourcing and strategy for support teams.
- Improved retention (to be measured the following year).
Due to the changes in the support structure, these benefits will not be realised this year. While the support teams now use a statistical basis to target student cohorts, the effectiveness of this was not able to be evaluated in a statistically sound fashion.
Un-anticipated benefits were realised as part of the project:
- Identification of most effective contact methods for improving student outcomes.
- Evidence of student outcome improvement for student contact teams.
- Recognition and quantification of at-risk cohorts (e.g. 18/yo female pre-degree students).
- Identification of a “cut-off” period of 5-6 weeks for most semester-length courses, after which student support services are less effective.