Real-world results include:
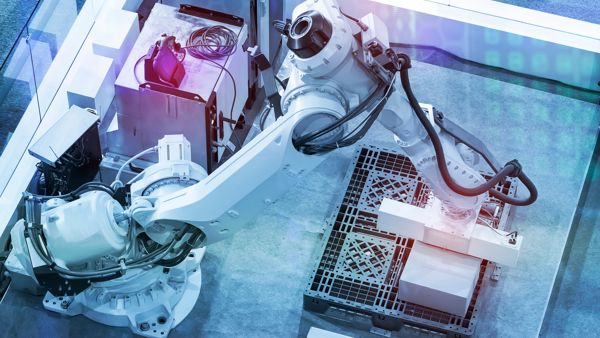
How factories are exploring the use of AI in their production lines
Manufacturers are under mounting pressure to do more with less. A minute of factory downtime can cost companies thousands of pounds, and increased delays can mean missing out on consumer demand at important moments like Christmas time or Black Friday. The ability to process and analyse huge volumes of manufacturing data using AI is already helping them to predict and prepare for potential disruption.
The Fourth Industrial Revolution, characterised by advances in automation, data analytics, connectivity and increasingly artificial intelligence, has had a profound impact on manufacturing, enabling companies to drive growth, improve productivity and overcome labour shortages.
For proof, look no further than the Global Lighthouse Network, a World Economic Forum initiative that shows how the Fourth Industrial Revolution (4IR) is reshaping production. This growing community spans every manufacturing sector across more than 150 global sites. Each demonstrates leadership in applying 4IR technologies to drive step-change financial, operational and environmental gains.
Lighthouse sites, on average, have realised double-digit improvements in productivity, efficiency and financial performances, workforce engagement, resiliency, speed to market and decarbonisation.
Increasing factory throughput by 200% while reducing resource use by more than 30%
Raising efficiency by more than 10% while cutting greenhouse gas emissions by 50%
Raising quality by 300% while reducing cost by more than 20% (World Economic Forum)
These outcomes are tantalising prospects for any manufacturer. No wonder investments in 4IR technologies, particularly AI, are happening at scale.
AI in Production Lines Adoption is Accelerating
AI’s integration into production lines is becoming more and more commonplace, with manufacturing solutions increasingly incorporating AI ‘out of the box’. Take automation, for example. The arrival of generative AI, a type of AI technology capable of creating something new after being taught something, is transforming human-machine interactions.
According to the International Federation of Robots, robot manufacturers have begun developing generative AI-driven interfaces that allow robots to be programmed more intuitively using natural language instead of code.
Digital twins, another core AI-powered 4IR technology, use real-time data, sensors, and computer simulations to mimic the behaviour and characteristics of physical components, processes or systems. As they exist solely in the digital domain, these virtual models can be stress-tested and modified without safety implications and with minimal cost.
AI is also revolutionising safety and efficiency in critical operations. For instance, the Abu Dhabi National Oil Company (ADNOC) produces three million barrels of oil a day. To refine that crude oil into petroleum, operations must be as efficient as possible. Every lost minute of production costs millions of dollars.
ADNOC has built and deployed AI-powered digital twins across thousands of plant assets. These systems provide real-time insights into asset performance and identify potential improvement opportunities, thereby maximising efficiencies, reliability, uptime and safety.
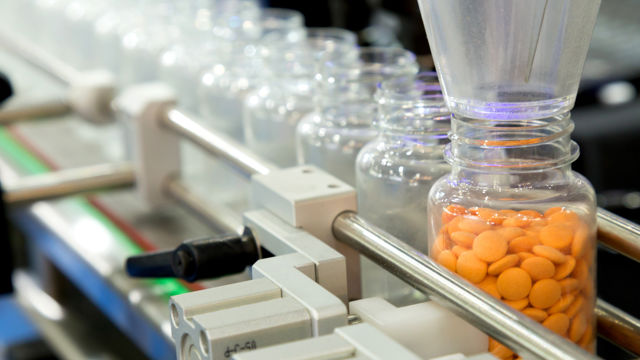
How AI is Reshaping Maintenance Strategies to Minimise Downtime
With downtime costs skyrocketing, artificial intelligence is helping prevent unexpected stoppages and propel predictive maintenance into the mainstream
Four and a half thousand miles away, AI is transforming waste management operations. London-based Recycleye uses AI technology with FANUC robots to automate the detection and sorting of mixed recyclable material, traditionally done by hand.
This AI-powered system operates 24/7, significantly boosting sorting accuracy and line output, while reducing manual labour requirements – a crucial advantage in a sector facing a shortfall of 89,000 jobs by 2028.
As factories become increasingly automated, digitised and interconnected, manufacturers face the challenge of how to manage and leverage these technologies and systems to their full potential. Many are finding that AI holds the answer.
AI is Supercharging Traditional Manufacturing Solutions
Contrary to common perceptions, artificial intelligence won’t replace your MES, PLCs or welding robots. Rather, it will enhance their capabilities and unify them. AI’s strengths in data processing, automation, machine learning and adaptive decision-making enable it to do this in ways no other solution can match.
Simplifying tasks to increase efficiency is a common theme for how AI is being incorporated into production tasks. Programming a computer numerical control (CNC) machine, for example, is a highly repetitive, time-consuming and manual process that has remained largely unchanged for decades. Until now.
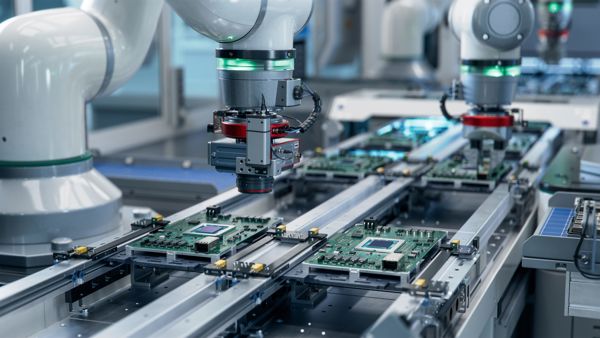
CAM Assist is a software tool that uses AI to understand (almost) every way a CNC machine could make a component, the rules of machining and the physics involved. When tasked with making a new component, it processes the potential appropriate methods and decides which combination would create the most efficient machining strategy. Doing so might require billions of complex calculations and could only be achieved using AI technology.
Developed by London-based CloudNC and refined in the company’s production facility in Essex, CAM Assist can produce reliable, professional toolpath instructions at the click of a button. It can complete up to 80% of the machining strategy, leaving the remaining 20% for manual review and adjustments.
This streamlined approach can save hundreds of production hours, enabling engineers to accomplish tasks in seconds that usually take hours or even days. Benefits to manufacturers include faster and more efficient CNC programming, accelerated staff training and a quicker, more accurate quoting process.
AI is helping make sense of endless data
The advent of 4IR has brought a tidal wave of data from sensors, machines and interconnected devices. The Industrial Internet of Things (IIoT) is a large contributor, continuously collecting and transmitting data from factory assets, equipment and infrastructure to create a digital thread throughout the production cycle.
While the sheer volume of data can feel overwhelming, it isn’t just noise. Real-time updates related to temperature, pressure, vibration, energy consumption and asset utilisation provide a rich source of information for optimising production lines and improving worker safety.
AI in production lines is helping make sense of endless streams of information by transforming raw data into actionable insights. It does so in four key ways:
- Data Collection and Integration
AI systems in production lines excel at collecting data from disparate sources, including production databases, sensors, machines and devices. This integrated data approach provides a holistic, 360-degree view of operations, enabling better, more timely decision-making across the factory floor. - Data Cleaning and Preprocessing
Raw data often contains errors, inconsistencies and irrelevant information. AI algorithms can clean and preprocess this data, ensuring its accuracy and relevance for analysis.
- Advanced Analytics
AI-powered analytics tools can analyse vast amounts of data at high speed, uncovering patterns, trends and anomalies that human analysts might miss. This capability is particularly valuable for predictive maintenance, quality control and process optimisation. - AI-Powered Automation
AI further streamlines operations by executing predefined tasks, workflows or responses based on predefined rules or machine learning models. This helps reduce manual intervention, minimises costly errors and accelerates decision-making processes.
Unleashing AI’s Full Potential into the Production Process
While AI is generating significant excitement, it’s important to maintain a balanced perspective. AI is not a universal solution and can’t solve every production setback or bottleneck single-handedly.
While AI can automate many aspects of data analysis, human oversight and domain expertise remain crucial. The most effective method often involves a human-in-the-loop approach, combining AI’s analytical capabilities with human judgement to validate insights, interpret results and refine AI models over time.
Furthermore, the quality of data significantly impacts the effectiveness of AI algorithms. Poor-quality data, such as missing values, inconsistencies, outliers, and inaccuracies can lead to biased or misleading conclusions.
Having mechanisms in place to ensure data quality through regular validation, cleaning and normalisation processes is essential. Understanding the context and business objectives is equally crucial in selecting and preparing the right data for analysis.
Establishing robust data governance practices, including documenting data sources, metadata and data lineage, alongside defining data quality metrics and standards, is key. Doing so ensures data integrity, reliability and relevance, laying a solid foundation for AI in the production process to unleash its full potential.
Read more of our from our AI in Manufacturing series...